AI in capital markets: hype, hero, or horror?

By Justin Hingorani, Head of Platform
AI is one of the hottest topics in financial services right now. It’s also a subject that’s close to our hearts; Duco customers have been using AI for years to expedite reconciliation setup and matching, saving them time, effort and cost.
As such, we were delighted to bring together several AI experts for an in-depth panel discussion during Customer Innovation Day. Joining me on stage to explore the possibilities, pitfalls and practicalities of AI were:
- Sanjeev Prabhakar-Badri, Principal, AWS Data, SaaS and Enterprise Software
- Mark Barton, CTO, Jaid
- Julian Beckers, Intelligent Automation Director, Ciklum
Read on to discover the key takeaways from our conversation, including the right way to use AI, the typical adoption path, how to overcome common concerns and the paradigm shift coming up for AI.
Hype or horror: is AI actually useful for capital markets firms?
I kicked things off by inviting Sanjeev to tackle one of the most common objections to AI: it’s all hype, isn’t it?
But Sanjeev shared a story about an AWS partner and asset manager with over $2tn AUM: “We had an executive briefing session with the Chief Data Officer and the Chief Operating Officer. They said they see 17 partners in their landscape as strategic. The board is getting to a view where if any of those 17 partners is not having a clear AI strategy, they probably will consider re-evaluating that partnership.”
Our audience polls on the day showed a lot of interest in AI as well. While it’s true that nearly 50% of attendees thought it would be more than a year before their business area deployed AI to solve a major problem, just under a third had either already done so, or expected to within 6-12 months.
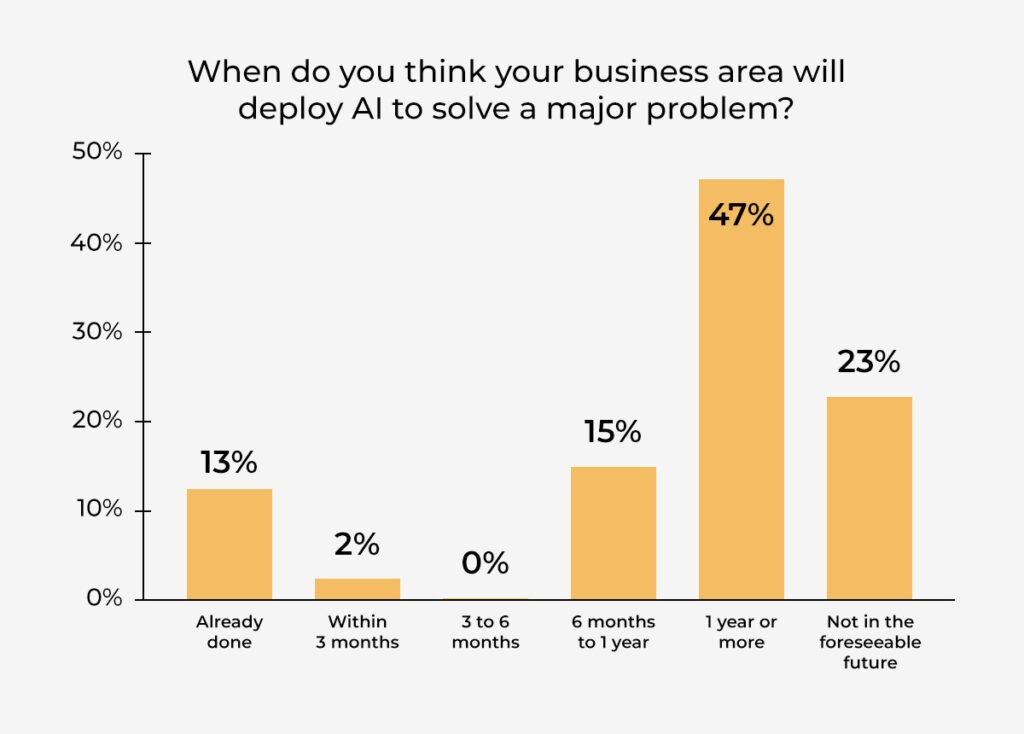
And the majority – 44% – felt their leaders were underestimating the impact AI can have, which may explain the slow outlook for adoption. Not even a quarter felt that their senior management team were overhyping the benefits of AI.
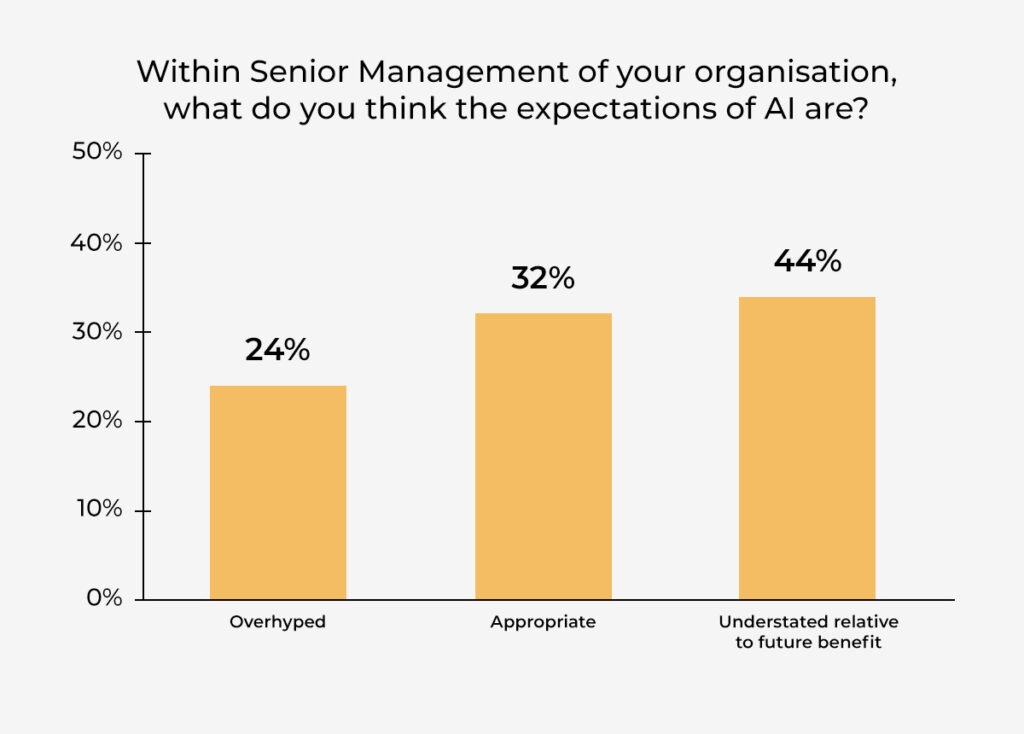
What this shows is that leading firms in the industry – your peers and competitors – are taking AI seriously. And for good reason. Cost and headcount savings are the most talked about, but the benefits of AI go far beyond that.
As Mark from Jaid pointed out, there are many ways AI can benefit capital markets. Talk often focusses on the risks AI supposedly introduces (more on this later), but the technology can actually improve processes that inherently carry risk. Mark explained that AI can perform repetitive tasks indefinitely without getting bored, or distracted. If implemented correctly, AI is consistent and therefore de-risks these processes.
On top of this, there are a wide range of tasks in capital markets that are so manual no one ever does them.
(We run into this a lot when talking to customers around their successes with the Duco platform. The most common response to the question ‘How were you doing this before Duco?’ is ‘We weren’t. It was too difficult or time-consuming’.)
AI can do in hours or days what it could take a human worker thousands of hours to achieve. Suddenly this makes an impossible task possible.
Sanjeev also shared a concrete example of a firm using AI to better support their teams. One AWS customer, a large bank, has a risk innovation team. Their job is to create risk manuals containing the various compliance and regulation-related information. They have started ingesting these PDFs to power an AI chatbot that can answer questions about various aspects of risk.
According to Sanjeev, “one of the field reps said ‘This is great because it’s like conversing with the risk manual’. They could leave natural language questions and get an answer back.”
One Duco customer trains an AI on all their internal documents, allowing anyone in the business to ask questions about policy and get an answer.
OK, so AI’s useful, but is it safe?
Julian from Ciklum noted that AI undeniably has some drawbacks and risks. However, he pointed out, so does driving your car in winter. And the debate around AI is similar to what was seen with other general purpose technology, such as electricity.
The technology is still coming of age and so problems are to be expected. One such issue is ‘hallucinations’. This is where the AI perceives patterns that are indiscernible or illogical to humans, producing results that are nonsensical or factual incorrect.
But, Julian says, there’s already a tech stack emerging to help combat these problems, whether by incorporating guardrails into the models themselves, or adding additional guardrails on top.
It’s also worth remembering the prediction from our CEO, Christian Nentwich, during his keynote to open the event: “All these things are going to be fixed much faster than everyone thinks.”
But is my data secure?
Ours is an industry that handles a lot of very sensitive, confidential data. Can you trust providers with your data?
In fact, information security was the top concern our audience had when it came to AI. This was followed closely by data quality and the challenge of integrating AI with legacy systems.
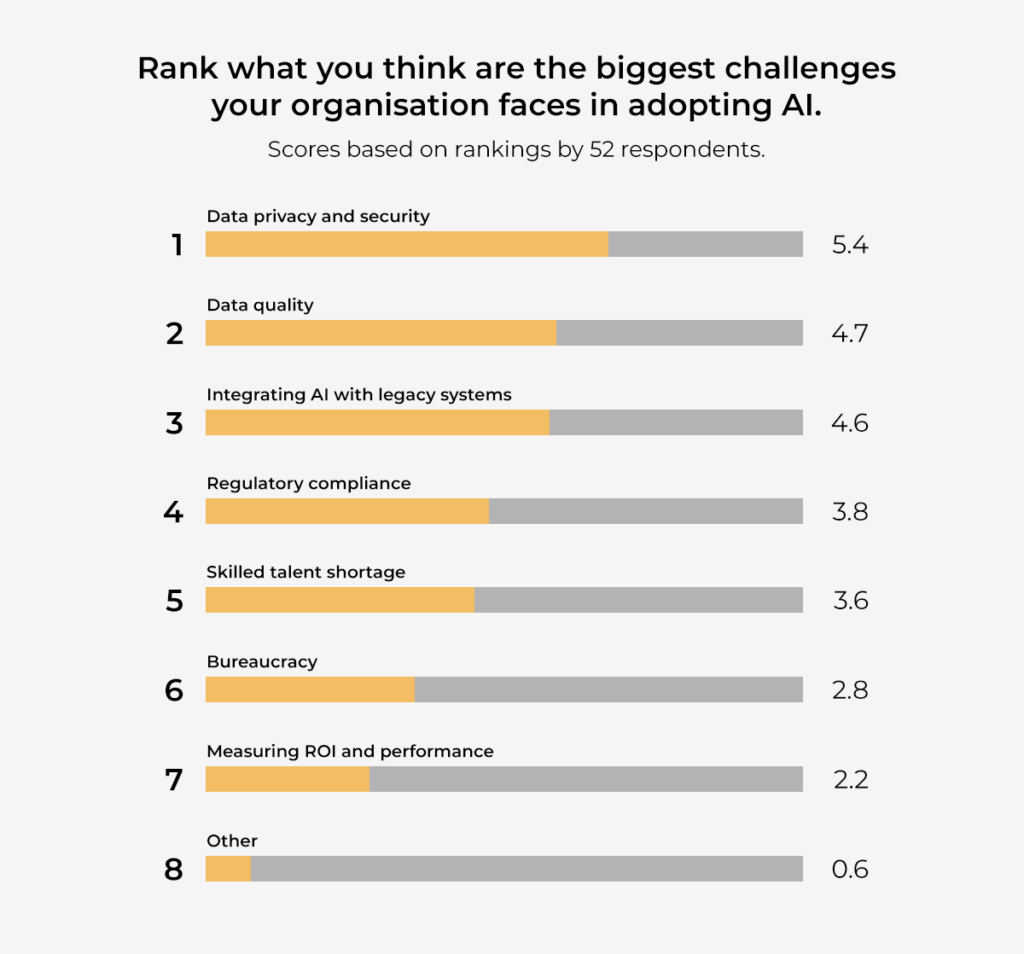
“Security concerns – there is not a single client we talk to where they don’t have information security going ‘Oh my god, we can’t put our very sensitive data on the cloud; how do we know you aren’t going to leak it?’ All those problems, that we all know exist,” explained Mark.
Yet once information security teams look into the platform and the service and understand what’s being done and how, they’re comfortable with it. And, as Mark put it bluntly, firms like Jaid couldn’t survive the reputational and financial repercussions if they ever leaked client data.
“It’s very different to give some data to somebody in a very secure environment for a very short period of time to train your model than it is to just open everything up to the cloud,” he said.
The AI adoption path
So how do most firms who are already leveraging the power of AI go about it?
According to Sanjeev, the typical place to start is by applying a generic large language model (LLM) to a particular use case. These models are available to AWS customers. This initial project often works quite well, but what customers begin to see is that as they broaden out the use cases the LLM stops performing as well.
That leads firms to explore smaller, more tailored models that they can train using their own data, for the purpose of just one, narrow use case. Business context is very important to help the model understand what kind of outputs it should be generating. AI models are not one-size-fits-all solutions. They are tools that excel when applied to a particular job. The difference is that the tools can be shaped to better suit the job in question.
And what does training, for example an LLM, for a use case look like? Julian explained that there are three stages firms go through.
The first is prompt engineering and behavioural adaptation. This is where you begin to identify what the model can already do given the data it was initially trained on and start generating outputs.
Next up you feed in your own data, in order to provide that business context that can fill in the gaps in the model’s own training data. The third stage is to fine-tune the outputs by further altering and improving the way the model behaves.
How do we ensure we’re using AI right?
As you’ve probably picked up on by now, one of the takeaways from the panel was that AI is not a panacea. It is a powerful tool when applied in the right way. But, as Julian said, if you give someone a hammer a lot of things start to look like nails.
“The most efficient way to use Gen AI would be for the category of use cases that it fits to very well,” he said. “And this is what we’re seeing the market is not doing properly. Using one of the foundation models to come up with a recipe is one thing; to do a credit scoring is another thing. These models are now a bit abused for use cases they were not meant to be for in the first place.”
So with that in mind, what are the right applications for particular forms of AI? How do you ensure that you are using it effectively?
The first point may seem obvious, but given the state of the general conversation around AI it’s worth repeating what our panellists said: use an AI for the use cases in which its abilities fit well.
Unstructured data is a great example. “Unstructured data is 80% of enterprise data”, Christian explained during his keynote. It is a big target for firms looking to improve their data automation, as we heard during our chat with Santander Chief Operating Officer Jose Munoz at Sibos last year.
Ingesting and understanding the PDF risk manuals that Sanjeev mentioned earlier is a great example of unstructured data. Scraping emails and providing key information in another format that can be easily loaded into other automation systems is another.
Our audience believed that AI would have a broad impact across all tasks associated with data automation. Organising and structuring topped the list, followed closely by transforming and reconciling data.
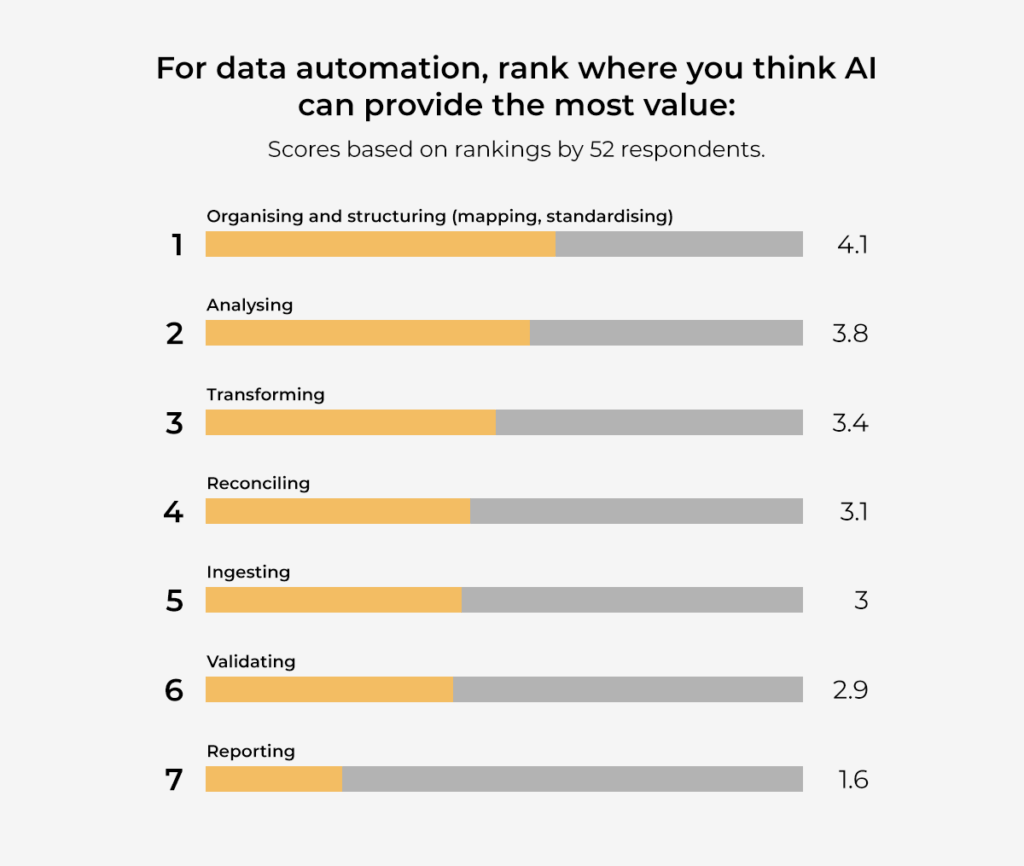
Also, make sure you’re on the cloud. While not being cloud-ready isn’t entirely a blocker, being on the cloud will give you a huge advantage. Cloud providers can offer far superior and scalable computing power to help train your models, giving you a time-to-value advantage. As Sanjeev said, “you don’t need to set up things; you have technologies available and you get going.”
The other point our panellists raised was the need to have strong governance in place. This is not only good for internal audit and understanding how your models are performing, but also because regulation is inevitable. According to Sanjeev, firms are largely positive on the notion of regulation for AI. They believe it will bring security, reliability and transparency. But at the same time there will be things they have to do to ensure they are compliant, so following best practice from the start is one of them.
What’s next for AI? Why Gen AI is such a step forward
Gen AI is a paradigm shift for artificial intelligence, Julian said. “Calling AI and Gen AI the same is like saying birds and planes are the same because they both fly and have wings.”
What Julian referred to as “AI 1.0” involves using statistical models for narrow use cases. They are able to automate tasks. What Gen AI can do is be applied much more broadly, solving a range of problems.
Sanjeev explained: “Gen AI helps you with reasoning. Human cognition of how you would interpret the next step, you can now automate that at scale using Gen AI. So reason plus tasks – automating that at scale can be really powerful. Productionising this is the next challenge.”
In terms of adoption, our polls suggest it could still be a little while before we start to see mass deployment of AI solutions, but there is already a significant portion of businesses that are experimenting with the technology and this number is only going to grow.
Conclusion
AI, like any emerging technology, is surrounded by hype and scaremongering. As usual, the reality lies somewhere in the middle.
While there are risks, there are ways to mitigate them; while the potential is massive, AI is not a solution for every problem.
But when you respect the power of the tool, use it for the right application, and put the same controls around it as you would any technology or process, it can be a significant driver of operational efficiency.
Some capital markets firms, including Duco customers, are already feeling the benefits.